AI in the Contact Center
Posted in M&A Insight
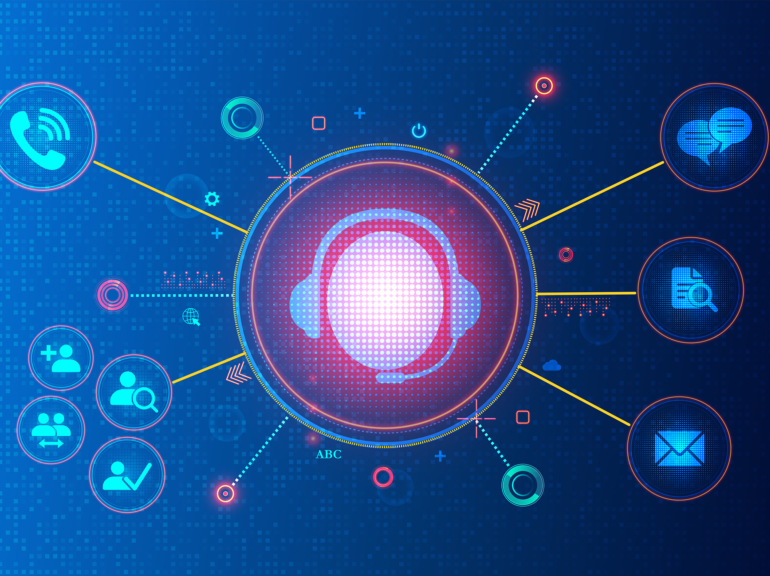
After several years of “AI hype,” we are now starting to see true AI deployments and results in the contact center. AI applications typically fall into one of three categories: agent supporting, virtual agents, and interaction insights and analytics. Most companies tend to start with digital and agent-facing applications before moving toward voice and customer facing channels that can be more complex. Beyond speech analytics, natural language and intelligent virtual agents (all of which have been implemented in contact centers for years), some examples of the types of AI deployments that we currently see bringing true value to the contact center include:
Agent Supporting
- “Next best” action and/or “best use” conversational resolution – the AI engine scans agent desktop actions and analyzes the agent/customer conversation to make suggestions to the agent for the next response, desktop input or KB search, saving both agent handle time minutes and customer time for issue resolution
- Automated documentation and interaction summaries – creating case notes in the CRM system based on the conversation and desktop interaction, again, saving costly agent handle time minutes (both during the call and in after call work)
- Reality modeling for training – enabling an agent to conduct an actual role play with the AI platform, during which the AI reacts as a customer based on the agent’s dialogue using many recorded examples of both successfully and unsuccessfully resolved conversations; this approach is much more effective than asking the class to pair up so learners can role play with each other, since the trainees are not subject matter experts and have no way of knowing if they if they are “handling” the role played call correctly
- Best agent modeling – creating training modules, scripts, and desktop interaction flows based on top performer activities, with the goal of reducing the new-hire agent learning curve and, potentially, decreasing training delivery time
Virtual Agent
- True conversational IVR/chat – with AI, the IVR interface moves from simple responses to more complex conversations with the customer, to include asking clarifying questions as needed, with the goal of containing the customer in the IVR if his or her request can truly be resolve in the self-service channel
- Automated email responses – creating email responses that are made up of various sections of text combined to deliver a customized and comprehensive reply that addresses each of the customer’s statements or requests, vs. the historically one-dimensional “canned” responses which usually result in another customer email or phone call to the company to resolve the issue(s)
Insights and Analytics
- Automated Call Monitoring – while speech analytics vendors have touted automated QA observations for years, the AI engine is the breakthrough that enables transformative QA monitoring for compliance, “hot topics” and trends, customer insights and even agent knowledge application, while delivering a sample size that is statistically valid
- Intent Analysis – a step beyond normal speech analytics applications that can evaluate voice or chat interactions to better understand customer intent and diagnose the root cause of customer-facing issues
- Predictive NPS – using voice inflection and language to assess customer sentiment and assign likely NPS ratings to each interaction based on previous contacts and corresponding post-interaction surveys